Table of Contents
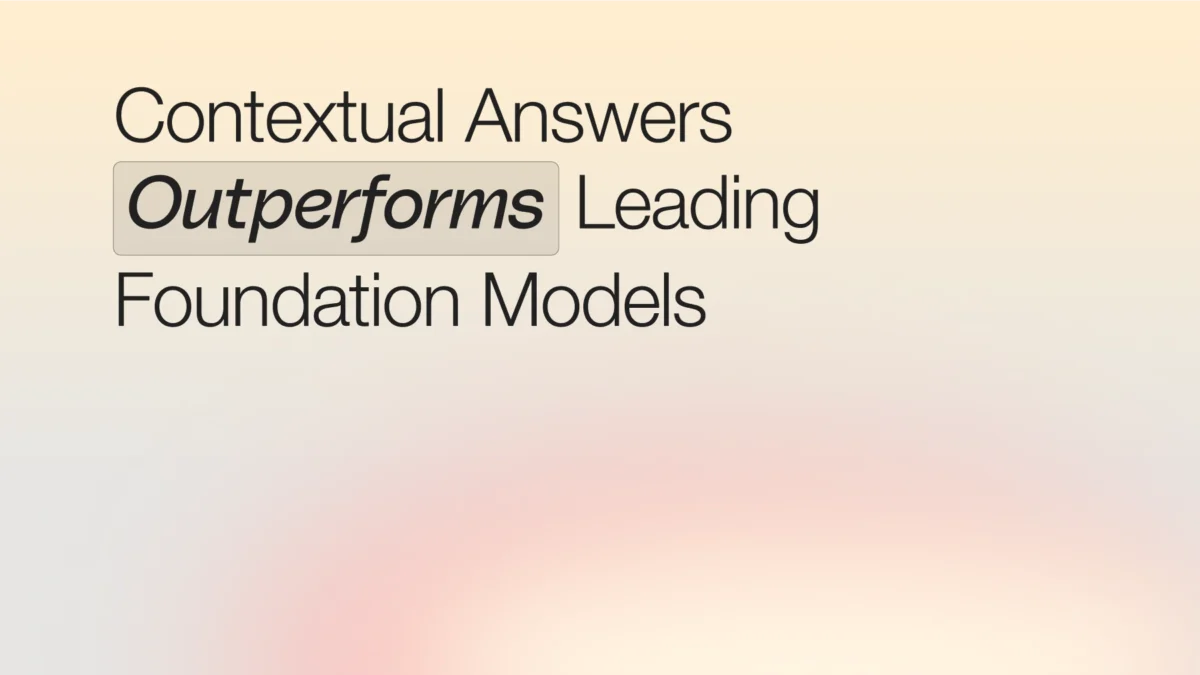
AI21’s Contextual Answers Outperforms Leading Foundation Models on Question Answering Tasks
Three important metrics determine the quality of a language model’s question answering capabilities: output accuracy, context integrity, and answer relevance. By those metrics we mean:
- Output accuracy: A model’s ability to identify both questions that can be answered using the given information and those for which there is no answer available.
- Context integrity: A model’s ability to minimize hallucinations by correctly claiming the context does not contain an answer.
- Answer relevance: The model’s generated answer sticks to the subject and constraints of the question, without elaborating with additional and unnecessary details.
Together, these three measurements indicate how reliable a model is, a critical factor for enterprises seeking to implement GenAI solutions. There is nothing more important than ensuring a model will not hallucinate, for example, when providing information to customers.
Therefore, at AI21 Labs, we conducted an experiment to measure the performance of our Contextual Answers model—a Task-Specific Model specifically built to excel on question answering—versus the most dominant Foundation Models on each of these metrics.
We found that Contextual Answers outperformed Claude 3 Sonnet and Haiku, GPT-4 Turbo, GPT 3.5 Turbo, and Mixtral 8x7B, positioning Contextual Answers as the most reliable model for question answering tasks among leading Foundation Models. Below, we will review the methodology employed in these experiments, as well as the results.
Testing for model reliability
To gather data for the experiment, we randomly drew 1K examples from the well-known academic benchmark SQuAD 2.0, a dataset which consists of a series of contexts, plus related questions and a short golden answer for each. Within the 1K sample, we ensured a 50-50 split between questions with and without an answer.
Using this sample, we performed the following three evaluations:
Output accuracy
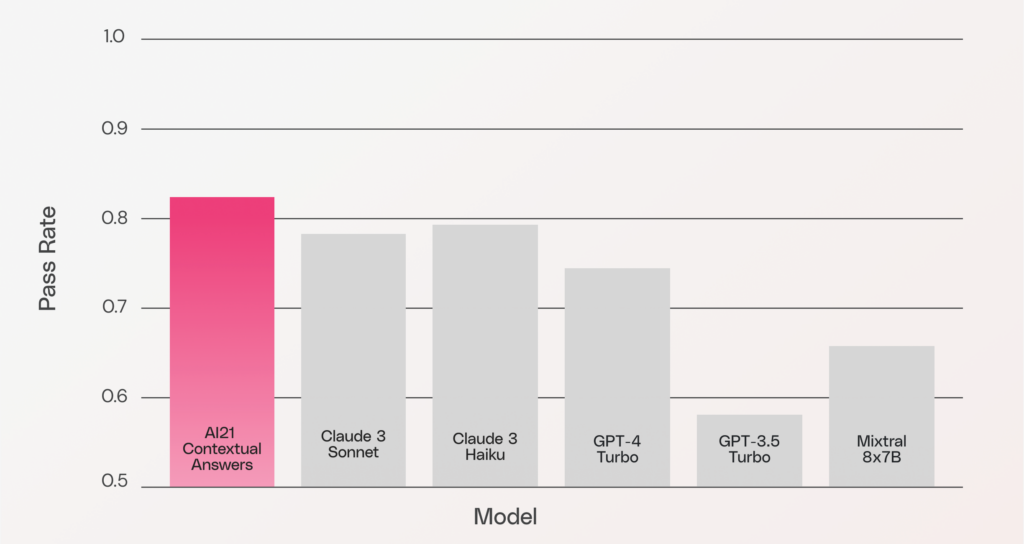
The pass rate is measured automatically using SQuAD 2.0’s standard metric. This metric takes into account both questions with answers and questions with no answer. AI21’s Contextual Answers outpaces the other Foundation Models in its ability to correctly identify when an answer can indeed be answered from the given context, and when the answer cannot be reliably found within the given text.
Context integrity
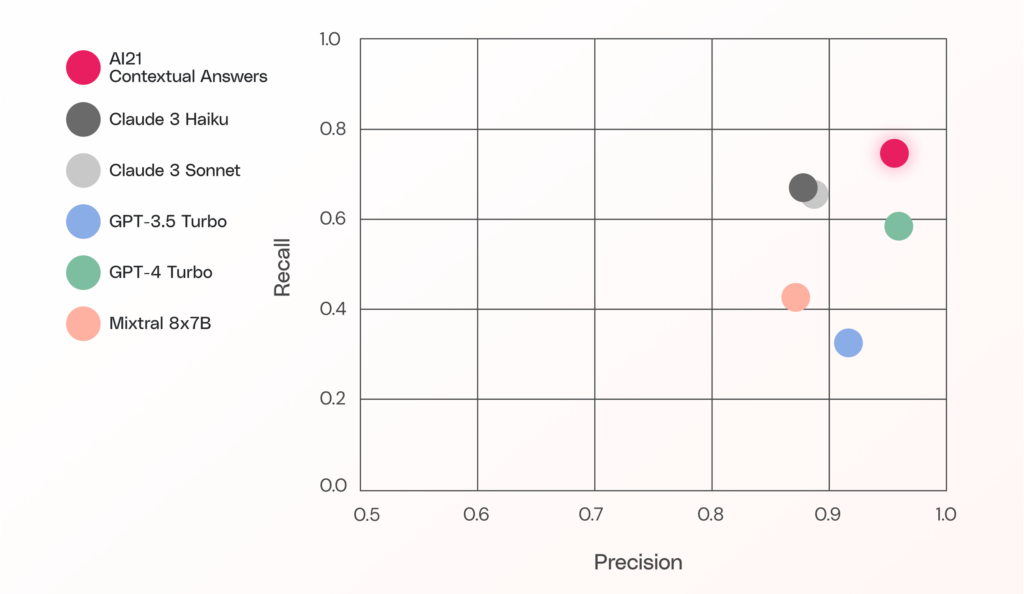
This experiment focuses specifically on a model’s treatment of questions without answers in the given context. The results are represented in a standard Precision/Recall graph, with precision understood as the model’s accuracy rate among questions it identified as unanswerable (i.e. from the total number of questions the model determined as unanswerable, how many are actually unanswerable questions?) and recall as the percentage of the total unanswerable questions the model was able to spot as unanswerable. As can be seen below, Contextual Answers leads the other models in its joint recall and precision scores, demonstrating its ability to avoid hallucinatory responses.
It is important to note that, although the prompts for all the Foundation Models included a specific phrase for the model to return in the case of an unanswerable question, we had to do extensive manual work to successfully catch all of those cases.
(Foundation Models (and especially chat models) have a tendency to provide answers which are fluent and with original phrasing, while, albeit very beneficial in conversation applications, do not lend themselves well to parsing automatic strings).
Answer relevance
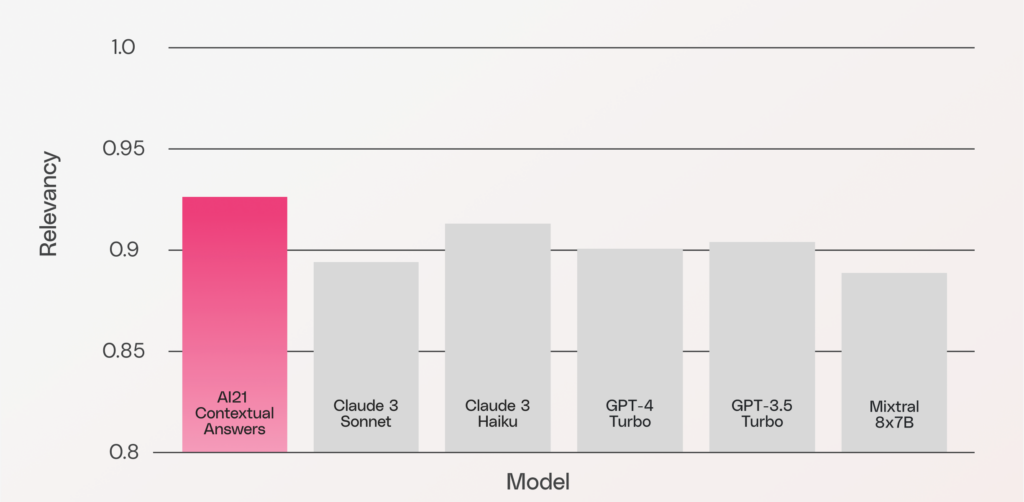
This metric measures how relevant a model’s generated answer is to the question. Importantly, this is solely a check of relevancy, as opposed to accuracy. Contextual Answers’ high score here indicates its strength at providing answers that are to the point, free from the embellishments of unnecessary details.
(Note: We used the RAGAS open source package for this metric).
Increased reliability with Task-Specific Models
Though large, general purpose Foundation Models are skilled at a wide range of tasks, small and specialized models can often outperform them when directed at the tasks they are built to solve.
As a Task-Specific Model optimized to excel at question answering, AI21’s Contextual Answers wins against top Foundation Models across multiple metrics of reliability, especially its ability to minimize hallucinations.
To learn how Task-Specific Models like Contextual Answers can bring value to your organization, speak to one of our GenAI experts.