Table of Contents
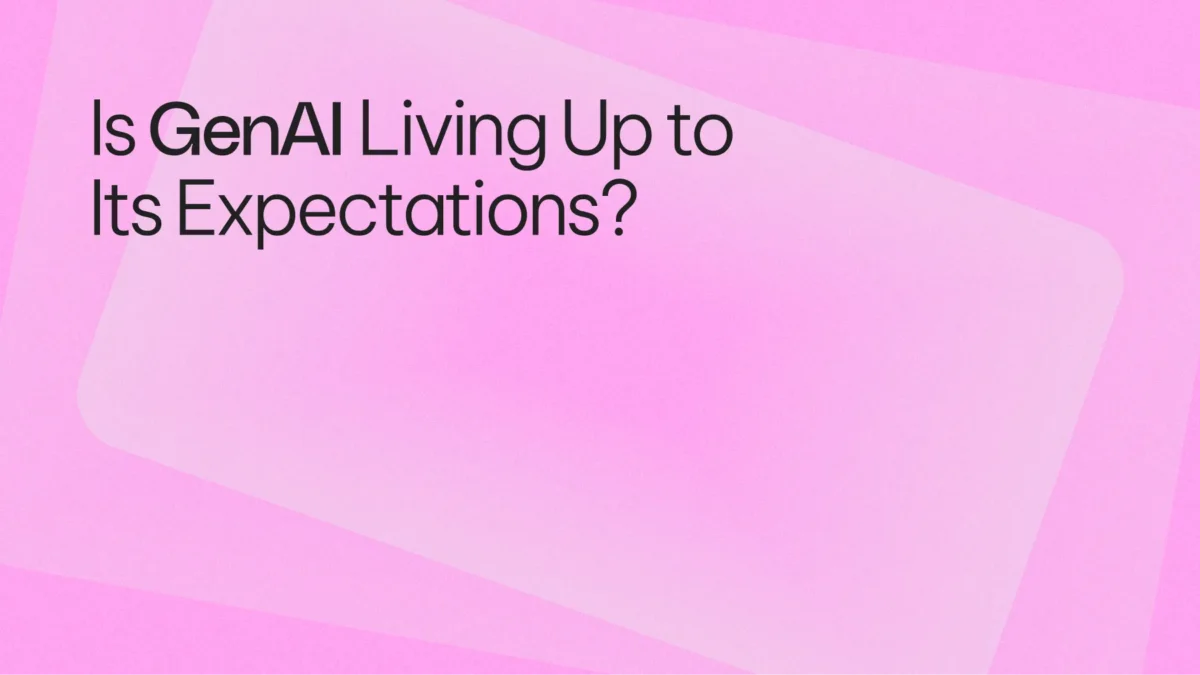
Is GenAI Living Up to Its Expectations? Insights from 3 Years in the Trenches
Remember when everyone thought GenAI would solve… well, everything? Yeah, about that. Three years into the GenAI revolution, we’ve learned some hard truths about what these models can (and can’t) do. Let’s cut through the hype and talk about where we really stand—because the next wave of AI innovation, with true agentic capabilities, is closer than you think.
The Not-So-Simple Present
Here’s the thing about enterprise GenAI: it’s like trying to build a race car while driving it. Sure, the technology is incredible, but scaling it? That’s where things get spicy. Initial implementations have revealed challenges we didn’t anticipate—from infrastructure costs running 40-60% higher than projected to integration timelines stretching 2-3x longer than planned.
At AI21 Labs, we’ve developed Jamba, a hybrid model combining the efficiency of Mamba’s state-space layers with Transformer attention’s precision. This architecture enables processing up to 256k tokens of context, redefining enterprise applications like financial document synthesis and long-form data analysis. However, it’s important to note that no single architecture solves all challenges—each comes with its own tradeoffs.
Jamba represents a pivotal shift in GenAI—one driven by hard-won lessons and deliberate design choices.
What Actually Works Right Now (And What Doesn’t)
The secret sauce for successful, accurate GenAI output isn’t one ingredient—it’s a recipe of multiple complementary approaches:
1. RAG (Retrieval-Augmented Generation)
Think of it as giving your AI an open-book test. By providing relevant information at inference time, RAG enables models to answer complex questions with greater precision. The more relevant context the model has, the better the output.
However, RAG implementations often struggle with:
- Data quality issues leading to inconsistent results
- High computational costs for large-scale retrieval
- Complex integration requirements with existing databases
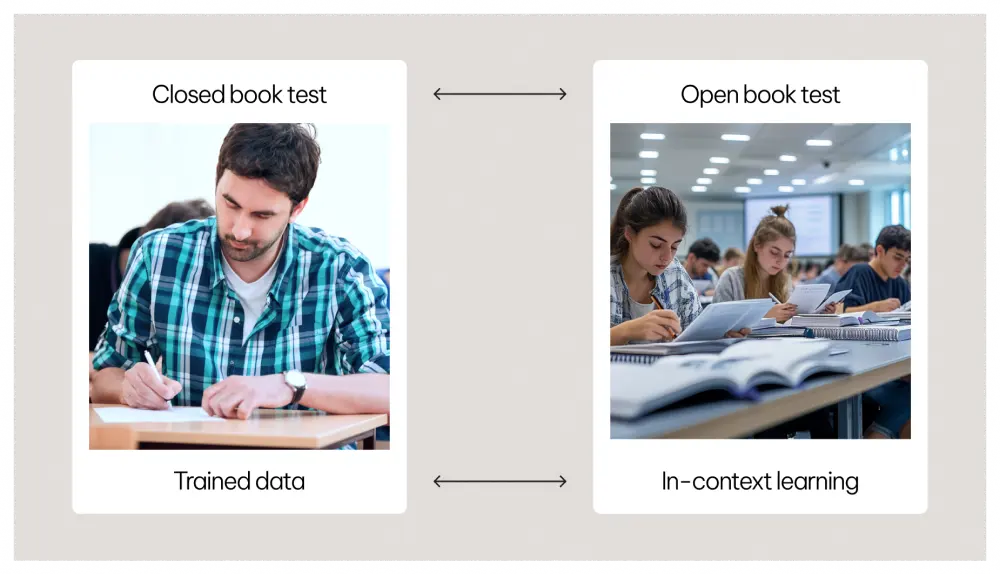
2. Systematic workflows
Breaking big problems into bite-sized chunks, early “agentic” workflows relied on rigid, rule-based “static chains” to ensure accuracy. AI21’s conversational RAG engine exemplifies this approach by enhancing output quality through step-by-step task construction.
- In building the RAG engine, but we’ve learned some hard lessons:
- Early “agentic” workflows were too rigid and brittle
- Error handling needs to be more sophisticated than initially thought
- Human oversight remains crucial for complex tasks
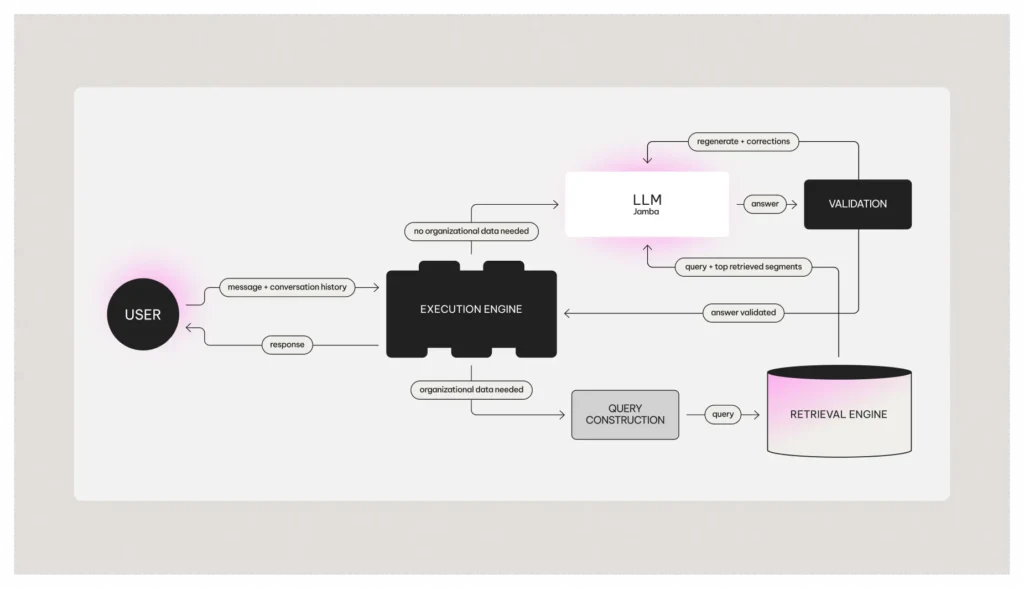
3. Prompt engineering
- This fancy term describes the art of “talking” to a model effectively, teaching your AI to speak your language. It’s a bit like being a model whisperer. Providing tailored prompts—and sometimes “few-shot” examples—can significantly boost performance.
While prompt engineering can significantly boost performance, it’s not a silver bullet:
- Results vary widely across different models
- Maintaining prompt libraries becomes increasingly complex
- Regular updates are needed as models evolve
4. Long-context processing
- Because sometimes you need the whole story. Why does long context matter? Databricks’ research showed that as context increases, so does accuracy. For real-world tasks, longer context windows are non-negotiable.
Longer context windows improve accuracy, but come with tradeoffs:
- Processing costs increase substantially
- Memory requirements can be prohibitive
- Not all tasks benefit equally from extended context
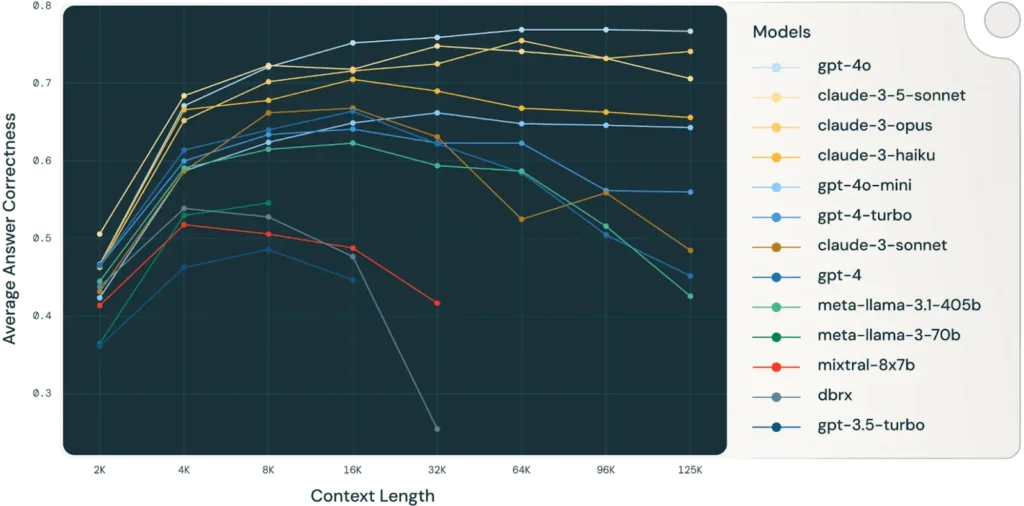
Combine these strategies above—past examples, a system approach for self-validation, and comprehensive context—and you’re feeding the model a mountain of data. Think about processing 10 years of Google, Meta, Amazon, and Microsoft’s 10-Ks. That’s 16,000 pages of context! Long context isn’t just helpful—it’s game-changing.
The Problem with Transformers
But here’s the catch: Transformer architectures dominate the GenAI market (e.g., GPT, Claude), but they hit a wall when processing very long contexts. Transformers are memory-hungry and painfully slow with large input sizes. Even models claiming to support long contexts rarely exceed 100k tokens in practice.
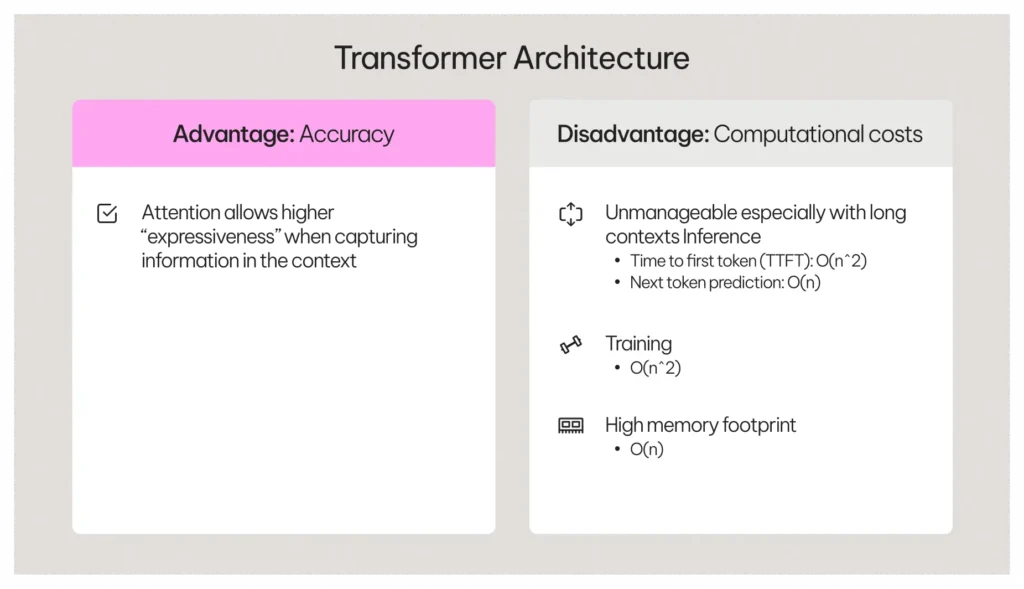
Those of you that played around with transformer-based models would also probably notice that even though some models have a declared long context window, they don’t really digest more than 100k tokens.
Enter Mamba, a state-space model and the first real alternative to Transformers. While more efficient, Mamba doesn’t quite match Transformer-level accuracy… which is where Jamba comes in.
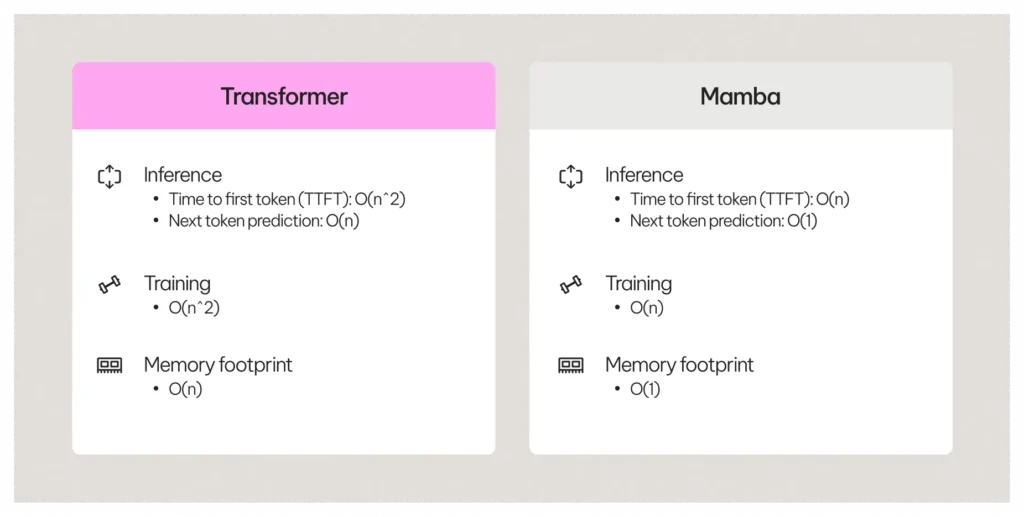
Jamba: The Best of Both Worlds
Jamba stands for Joint Attention and Mamba. It’s our novel architecture that combines:
- Transformer Attention Layers for unparalleled accuracy.
- Mamba Layers for efficiency and throughput.
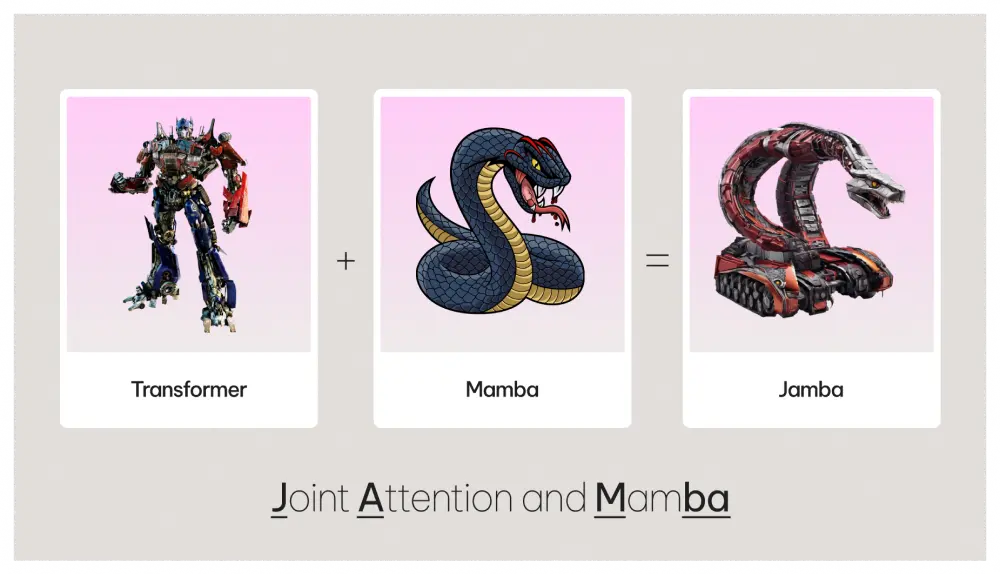
Each Jamba “block” includes seven Mamba layers and one attention layer—striking the perfect balance between quality and scalability. You’ll see below that in NVIDIA’s RULER benchmark, which measures effective context length, Jamba set a new standard. Our models excel up to 256k tokens, far beyond competitors.
Why this matters: With long-context capabilities, Jamba empowers use cases like:
- Parsing financial reports across thousands of pages.
- Synthesizing historical data for advanced insights.
The Long Context Benchmark: NVIDIA’s RULER
NVIDIA’s RULER benchmark tests how well models handle long contexts. It replaces older evaluations like “needle in a haystack,” which focused on spotting random strings in vast text but didn’t measure meaningful comprehension of large contexts.
RULER evaluates the effective context length of models—how much data they can actually process and use. While the benchmark stops at 128k tokens, our internal tests show that Jamba is the only model capable of reaching 256k tokens.
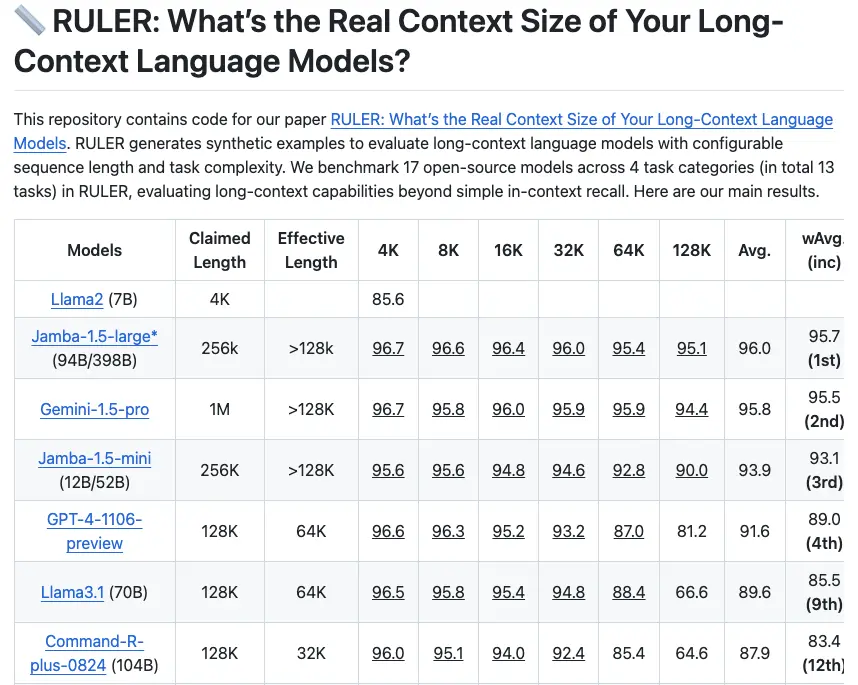
The GitHub leaderboard says it all: Jamba Large holds the top spot, with Jamba not far behind in third.
Critical Lessons from the Field
What We Got Wrong
- Initial Cost Projections – Infrastructure costs typically exceeded estimates by 40-60%
- Implementation Timelines – Depending on a company’s data science maturity, projects sometimes took 2-3x longer than planned
- Skills Requirements – Some team training required 6+ months instead of the expected 6-8 weeks
- Data Preparation – Consumed 60% of project timelines, far more than anticipated
What We Learned
1. Technical Reality Check
- Starting with GenAI is easy, but scaling it for enterprise use is much harder than expected
- Standard off-the-shelf language models are rarely ready for enterprise use – they’re both expensive to run and not accurate enough
- Successfully deploying GenAI requires deep technical expertise in system architecture and optimization
2. The Power of Chat Interfaces
- The game-changer was moving from basic AI models to conversational “chat” interfaces
- This shift made GenAI much more user-friendly and accessible
- More people could start using GenAI without needing technical knowledge
3. Enterprise Deployment Needs
- Companies need flexible options for where they run GenAI (cloud, their own servers, or a mix)
- Security concerns often mean building custom infrastructure
- Cost management is crucial – both for processing power and API calls
- Companies must optimize resource usage to keep costs reasonable
4. Getting Accuracy Right
- One accuracy check isn’t enough – you need multiple layers of validation
- The more context you can give the model, the better it performs
- Using multiple AI models together helps reduce errors and biases
- Regular testing and validation are essential for maintaining quality
Real-World Use Case: Financial Term Sheet Generation
Term sheets are a perfect example of tasks ripe for automation. Bank employees handle them daily—repetitive, standard documents requiring basic information extraction and formatting. It’s tedious, time-consuming, and low-value work. Automating this process with a long-context model like Jamba saves time, boosts accuracy, and lets employees focus on more impactful tasks. Here’s how to make it happen.
Start with Core Design Values
Building a GenAI solution means focusing on these critical principles:
- User Control: I want to have the flexibility to refine outputs.
- System Approach: To increase accuracy, I want to be able to pick the right tool for the right task.
- Contextual Input: I’ll supply all relevant data during inference to enhance model performance.
With these values as a foundation, let’s dive into how it comes together.
Step 1: Contextual Input and Key Points
- Input Data: Start with raw inputs like speech-to-text transcripts or other records.
- Human-in-the-Loop: Use Jamba Mini to extract and validate key points from the input. These validated key points will also serve as anchors for accuracy checks later.
Step 2: Generation with Tailored Prompts
The generation agent handles two main processes:
- RAG for Few-Shot Prompts: Instead of retrieving data for direct use, employ Retrieval-Augmented Generation (RAG) to curate examples of relevant term sheets. This step ensures that the model adopts the right tone, structure, and context for the task.
- Generation with Context: Combine input data, key points, and tailored examples to generate the first draft of a term sheet. This ensures the model has all relevant context at inference time, leading to precise and tailored outputs.
Step 3: Validation for Quality Assurance
Validation is critical to ensure the output meets high standards.
- Key Point Verification: Cross-check the generated output against the validated key points.
- Independent Critique: Use a separate model, like Claude, to act as a judge, providing feedback on the generated content. This multi-model approach reduces biases and improves reliability.
Outcome
The result is a high-quality term sheet generated faster and with built-in accuracy checks.
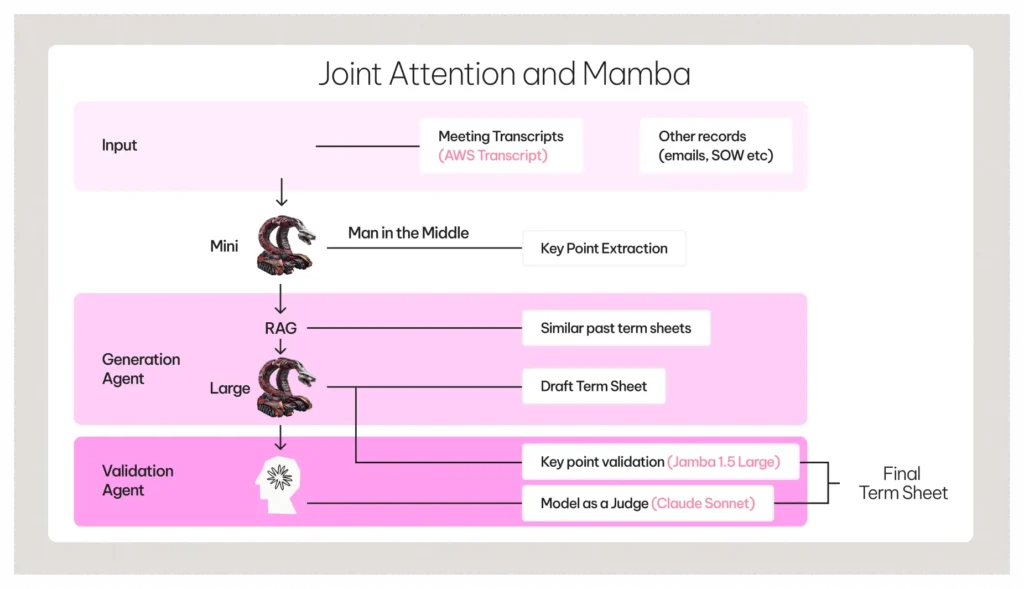
Proven Success in the Real World
We’ve deployed a similar solution for a leading international bank, automating term sheet generation while maintaining strict accuracy and compliance standards. These same design patterns are now applied across other use cases, proving the flexibility and scalability of Jamba.
Seamless Deployment Anywhere
One of Jamba’s standout advantages is its open-source foundation, allowing for deployment wherever it’s needed—on-premise, in the cloud, or hybrid setups. This flexibility ensures security and control for enterprise users, and Jamba’s superior benchmarks reinforce its value.
Jamba helps you build powerful GenAI applications by combining deep expertise, smart design, and flexible deployment that works at enterprise scale. Ready today for your needs, built for tomorrow’s autonomous AI.
The Next Frontier: Agents
The future of GenAI lies in agentic workflows—dynamic systems capable of adapting to complex tasks. Unlike today’s rigid, sequential automations, true AI agents will:
- Handle long context to dynamically adapt to new information.
- Explore different courses of action autonomously.
- Learn from failures and pivot in real time.
For this vision to become reality, models need very long context windows and the ability to integrate multiple tools and information sources. The technology is evolving, but we’re just scratching the surface.
Now while the future of GenAI lies in agentic workflows, we must be realistic about current limitations:
- True autonomy remains a significant challenge
- Error recovery capabilities are still primitive
- Integration across tools and systems needs improvement
- Cost and resource requirements can be prohibitive
The Road Ahead
GenAI’s early hype painted a picture of limitless possibilities. In practice, the journey has been more measured. Accuracy, scalability, and dynamic adaptability remain challenges—but we’re making strides.
GenAI’s early hype painted a picture of limitless possibilities. In practice, the journey has been more measured. While we’re making significant progress in areas like accuracy, scalability, and dynamic adaptability, important challenges remain:
- Infrastructure costs need to decrease further
- Integration processes must become more standardized
- Security and compliance frameworks need to mature
- Team training and development requires ongoing investment
Jamba’s success showcases what’s possible when you combine novel architectures, thoughtful design, and enterprise-grade execution. The technology isn’t a magic solution, but when implemented thoughtfully, with realistic expectations and proper planning, it can deliver significant value. And as we step into the era of agents, the best is yet to come.
GenAI isn’t just living up to expectations—it’s redefining them.