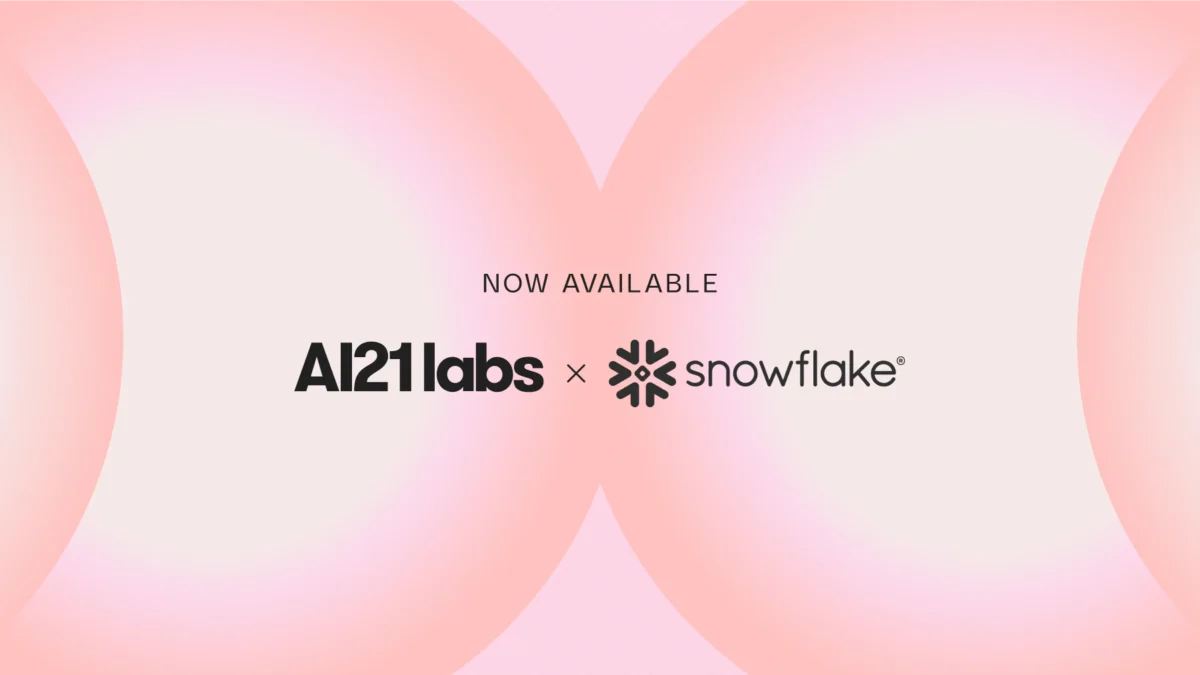
Analyze Long Documents Easily with AI21’s Jamba-Instruct and Snowflake Cortex AI
Today, Snowflake and AI21 are excited to make Jamba-Instruct, AI21’s groundbreaking foundation model that can process long context prompts effectively without sacrificing state-of-the-art performance, available for serverless inference in Snowflake Cortex AI. AI21’s Jamba-Instruct is capable of processing up to 256K tokens, enabling Snowflake Cortex AI customers to summarize or extract answers from content with roughly 800 pages of text documents in a single inference call. With this integration, Snowflake customers can instantly access Jamba-Instruct within their secured and governed Snowflake AI Data Cloud using a simple SQL statement.
AI21’s Jamba-Instruct has an industry leading context window, allowing customers to process large volumes of content at once. For context, 800 pages of text documents is equivalent to 8 years’ worth of a company’s 10-K filings, 32 earnings call transcripts, or 25 one-hour clinical trial interviews. This context window opens up use cases such as long document summarization, call transcript analysis, information extraction and reasoning over long documents and makes many-shot prompting possible. But what is really remarkable about AI21’s Jamba-Instruct is that it processes long contexts without a loss in quality and accuracy, a problem traditional transformer-based LLMs face as context length increases.
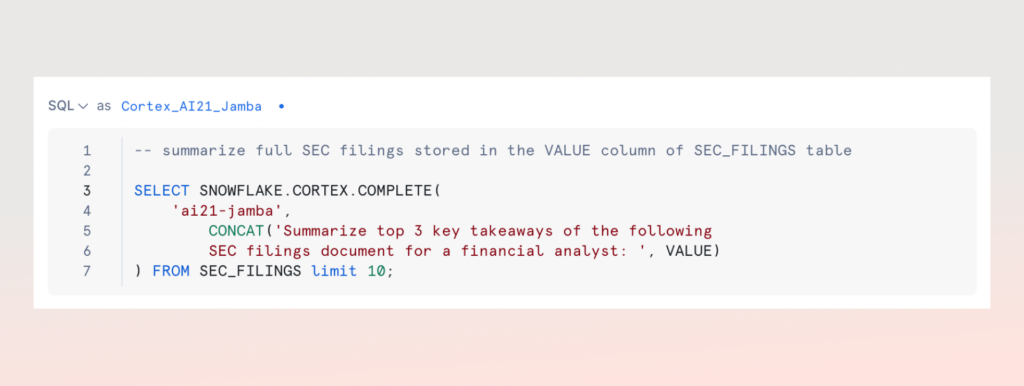
Snowflake Cortex AI is a fully managed service that gives customers instant access to serverless generative AI capabilities. With Cortex AI, users of all technical levels can securely create custom chat experiences, fine-tune best-in-class models, and accelerate no-code AI development on their Snowflake data.
The most recent model from AI21 Labs, Jamba-Instruct is the world’s first-ever commercial LLM to successfully scale up a novel SSM-Transformer architecture. Due to its hybrid build, Jamba-Instruct delivers leading quality, performance, and cost—offering one of the best values per cost across an impressive 256K context window.
Jamba-Instruct’s performance and efficiency and Cortex AI’s simplicity and security remove the common barriers to long context use cases—cost, complexity, and output quality— making it easier for enterprise customers to choose the model that best fits their specific use cases.
For developers and analysts looking to build with Jamba-Instruct on Snowflake Cortex AI, we’ve outlined the importance of a long-context window.
Importance of Long Context for Enterprises
Jamba-Instruct has the ability to handle more text and offer more room for instruction, improve output quality and simplify processes for many in-context learning language tasks.
One of the primary benefits of a long context LLM is the way it can help organizations tap into valuable information stored in lengthy documents. For example, financial analysts in investment banks or hedge funds can drastically improve their efficiency by using a long context LLM to perform tasks like summarization, QA, insight retrieval, and named entity extraction on lengthy documents like 10-K filings, which can regularly reach 100 pages each.
Other industries such as healthcare and life sciences, manufacturing, legal, and even HR, have similar opportunities to leverage LLMs to accelerate analytic tasks over long documents, such as conversational transcripts, contracts, or medical research reports and clinicians’ notes.
Another main benefit of a long context window is its ability to simplify complicated, advanced techniques often required to make LLMs performant. RAG is commonly used to ground LLMs in a customer’s vetted data and knowledge base; with a long context window plus RAG, a more accurate answer with a simpler architecture is possible. For example, when the relevant information is scattered across a long document or transcript, or across multiple documents, most RAG based solutions need to become significantly more complex. With a short context window, in order to return the right context to the LLM, you need an advanced, multi-step RAG architecture that can find and combine multiple chunks. Jamba’s long context window allows a single large chunk to be retrieved, simplifying the RAG pipeline while achieving excellent performance.
Finally, a long context window allows for many-shot prompting, an advanced technique that improves output quality by using repetitive prompts to guide the LLM to generate output in a desired style or format. One such use case is generating long product descriptions with a specific brand tone or style. Customers often do not have sufficient high quality training data to achieve a desired style via fine tuning. With a long context window, the user can feed Jamba multiple examples that illustrate their preferred style, guiding Jamba to tailor its outputs to match their brand’s style and tone.
Try it today
Jamba-Instruct in Snowflake Cortex is generally available to all Snowflake customers today at no cost through August 15. Check out our guided use case on Medium and if you’d like to learn more and discuss use cases for your own enterprise data, let’s talk.