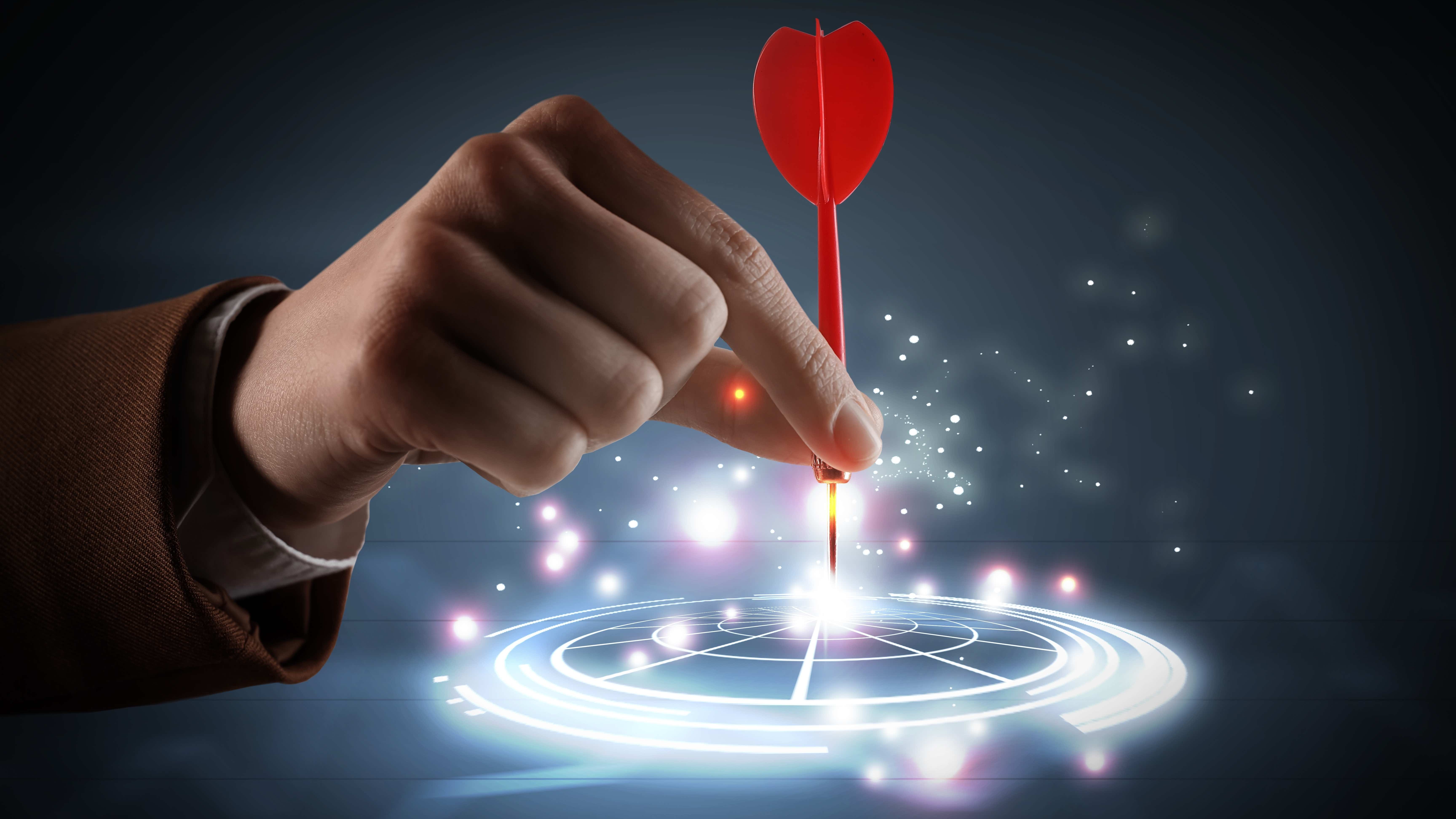
Grounding Language Models In-Context: Improving Text Generation and Attribution for Off-the-Shelf LMs
Text generation via language models (LMs) is dramatically improving, but LMs do not attribute their generated text to its sources and can often make mistakes. We propose the simple framework of ๐๐ฏ-๐๐ฐ๐ฏ๐ต๐ฆ๐น๐ต ๐๐ฆ๐ต๐ณ๐ช๐ฆ๐ท๐ข๐ญ ๐๐ถ๐จ๐ฎ๐ฆ๐ฏ๐ต๐ฆ๐ฅ ๐๐ข๐ฏ๐จ๐ถ๐ข๐จ๐ฆ ๐๐ฐ๐ฅ๐ฆ๐ญ๐ด, which allows for grounding ๐ข๐ฏ๐บ ๐ฐ๐ง๐ง-๐ต๐ฉ๐ฆ-๐ด๐ฉ๐ฆ๐ญ๐ง ๐๐ in knowledge from external sources, and attributing the text it generates to its sources.
โ
Recent advances in language modeling have dramatically increased the usefulness of machine-generated text across a wide range of use-cases and domains. An outstanding Achillesโ heel of LM generated text is that it is not attributed to a specific source, and often includes factual inaccuracies or errors. This problem is present in any LM generation scenario, and is exacerbated when generation is made in uncommon domains, or when it involves up-to-date information that the LM has not seen during training. A promising approach for addressing this challenge is Retrieval-Augmented Language Modeling (RALM), grounding the LM during generation by conditioning on relevant documents retrieved from an external knowledge source.
Leading RALM systems introduced in recent years tend to be focused on altering the language model architecture, and the need for changes in architecture and dedicated retraining has hindered the wide adoption of such models. Thus, while the RALM approach bears potential to alleviate factual inaccuracies and to provide direct sources for the generated text, it is in practice not deployed alongside leading LMs.
In our paper, we present In-Context RALM: a simple yet powerful RALM method which can be used for endowing any off-the-shelf LM with access to external knowledge sources. In-Context RALM simply inserts the retrieved document to a regular LMโs input, rendering it applicable even for LM behind API . While existing works choose which documents to show the LM via standard general purpose approaches, we propose several novel methods for grounded generation oriented document selection.ย
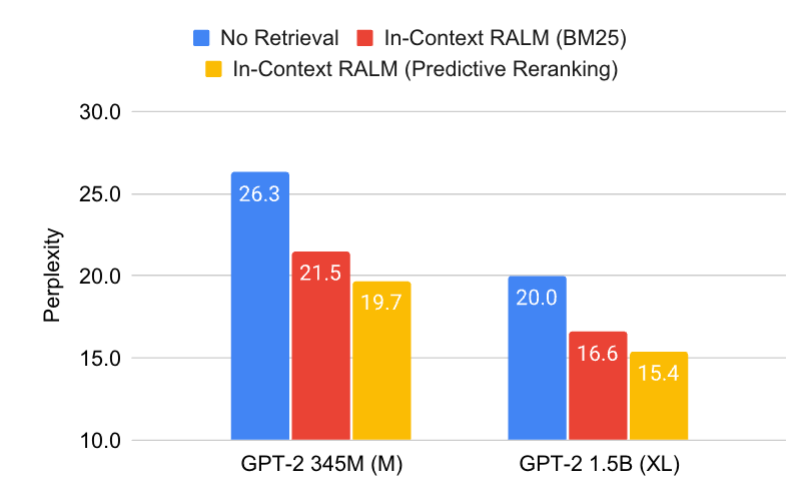
โ
Our simple and easily deployable setup allows improving the language modeling abilities of off-the-shelf LMs to those equivalent to increasing the LM's number of parameters by 4X, across a diverse evaluation set of five text corpora. We believe that further gains can be achieved via developing the generation-oriented retrieval mechanism, while retaining the straightforward document insertion mechanism of RALM.
To help others both to deploy and to build upon our work, our paper is accompanied by an online release of all our code, datasets, trained models, and indexes for our standardized suite of corpora.ย
Enjoyed this?
Stay up to date with the latest research and updates from AI21 Labs.